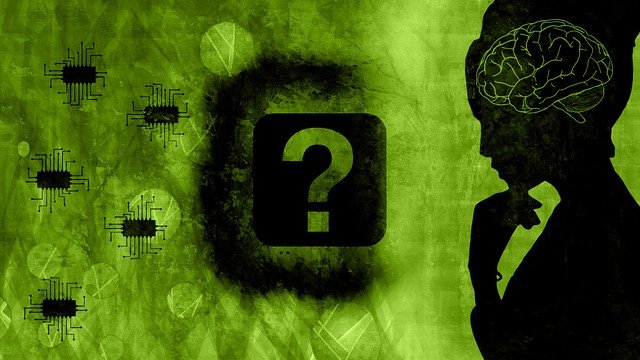
Our RAN automation solution
The left side of Figure 3 illustrates how the task of efficiently operating a RAN to best utilize the deployed resources (base stations or frequencies) can be divided into different control loops acting according to different time scales and with different scopes. A successful RAN automation solution will require the use of AI/ML technologies [6] in all of these control loops to ensure functionality that can work autonomously in different deployments and environments in an optimal way.
The two fastest control loops (purple and orange) are related to traditional RRM. Examples include scheduling and link adaptation in the purple (layer 1 and 2) control loop and bearer management and handover in the orange (layer 3) control loop. Functionality in these control loops has already been autonomous for quite some time, with the decision-making based on internal data for scheduling and handover in a timeframe ranging from milliseconds (ms) to several hundred ms, for example. From an architecture perspective, these control loops are implemented in the RAN network function domain shown in Figure 3.
The slower control loops shown on the left side of Figure 3 represent network design (dark green) and network optimization and assurance (light green). In contrast to the two fast control loops, these slower loops are to a large degree manual at present. Network design covers activities related to the design and deployment of the full RAN, while network automation covers observation and optimization of the deployed functionality. Network optimization and assurance is done by observing the performance of a certain functionality and changing the exposed configuration parameters to alter the behavior of the deployed functionality, so that it assures the intents in the specific environment where it has been deployed. From an architecture perspective, these control loops are implemented in the RAN automation application domain [7].
The green control loops encompass the bulk of the manual work that will disappear as a result of RAN automation, which explains why AI/ML is already being implemented in those loops [8]. It would, however, be a mistake to restrict the RAN automation solution to just the green control loops. AI/ML also makes it possible to enhance the functionality in the purple and orange control loops to make them more adaptive and robust for deployment in different environments. This, in turn, minimizes the amount of configuration optimization that is needed in the light-green control loop.
While the control loops in Figure 3 are all internal to the RAN domain, some of the functionality in a robust RAN automation solution will depend on resources from other domains. That functionality would be implemented as part of the RAN automation application domain. The RAN automation …….